Machine Learning Can Predict Rapid Kidney Function Decline
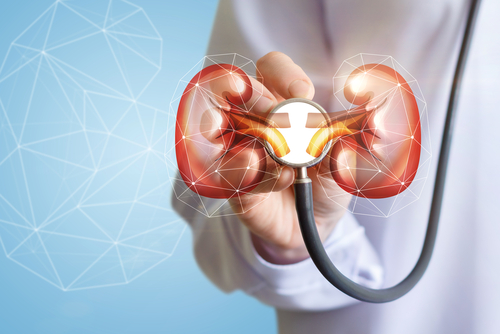
Machine learning tools can identify sickle cell disease (SCD) patients at high risk of progressive kidney disease as early as six months in advance, a study shows.
The study, “Using machine learning to predict rapid decline of kidney function in sickle cell anemia,” was published in the journal eJHaem.
Chronic kidney disease is more prevalent in SCD patients, who experience a faster decline in kidney function compared with the general population. As rapid kidney function decline in patients is associated with increased mortality, identifying those at risk early may help with timely use of preventive interventions.
Machine learning is a computer-based strategy that uses algorithms and statistical models, which learn from experience, to assess relationships between multiple variables, identify patient groups with similar patterns, and make predictions.
In the study, researchers at the University of Tennessee Health Science Center and their colleagues used machine learning tools to predict rapid kidney function decline in adults with SCD.
Kidney function decline, using data extracted from patient medical records, was determined using the estimated glomerular filtration rate (eGFR), a measure of creatine in the blood based on age, body size, and gender.
Creatinine is a metabolic waste product normally filtered by the kidneys. With declining kidney function, creatinine can build up in the blood and, to a lesser extent, in the urine. A normal eGFR is 90 mL per minute per 1.73 meters squared of body size per year (mL/min/1.73 m2 per year) or higher.
The team analyzed two groups of adult patients, making up an internal and external group. The internal group included 236 patients (133 women and 103 men, with a mean age of 31.15 years), who were followed for a mean period of 4.9 years.
In the internal group, rapid kidney function decline — an eGFR loss of 3 mL/min/1.73 m2 per year or greater — occurred in 166 (70.3%) patients. A severe kidney function decline — an eGFR loss of 5 mL/min/1.73 m2 per year or greater — was found in 140 (59.3%) patients.
The external group included 168 patients (86 women and 82 men, with a mean age of 32.5 years), who were followed for a mean period of 7.2 years. Rapid eGFR decline was observed in 145 (86.3%) patients, while a severe decline was seen in 136 (80.9%).
Data used in machine learning analysis included blood creatinine, hemoglobin, immature red blood cell (reticulocyte) count, history of stroke, diabetes, blood pressure, weight, age, sex, eGFR, and medication use.
Machine learning analysis of the internal group revealed that a rapid decrease in eGFR could be predicted six months in advance, with an 82% sensitivity (the test’s ability to correctly identify patients at risk) and an 80% accuracy (proportion of true results, both true positive and true negative).
Severe eGFR decline was predicted six months in advance with 79% sensitivity and 84% accuracy.
Machine learning tools also predicted a rapid eGFR reduction one year in advance with 53% sensitivity and 70% accuracy. Severe eGFR decline could be estimated with 64% sensitivity and 65% accuracy 12 months in advance.
In participants who had at least two consecutive eGFR measures of 3 mL/min/1.73 m2 or greater, at six months, rapid kidney function decline was estimated with a sensitivity and accuracy of 78%. In these patients, severe kidney function decline could be predicted six months in advance with 77% sensitivity and 81% accuracy.
The model predicted a rapid eGFR reduction one year in advance with 45% sensitivity and 67% accuracy, and a severe decline with 63% sensitivity and 65% accuracy.
The model’s ability to predict a rapid eGFR decrease six months in advance in the external group was similar to the internal group. However, its ability to estimate a severe eGFR decline six months in advance was poorer in the external group compared with the internal group.
After adjusting for disease features, age, initial eGFR, and changes in eGFR six months previously, reticulocyte count and systolic blood pressure were all associated with an eGFR decline of 3 mL/min/1.73 m2 or greater at six months.
Age and eGFR changes in the previous six months strongly predicted severe and rapid eGFR losses greater than 5 mL/min/1.73 m2. Initial eGFR, age, eGFR changes, systolic blood pressure, and blood creatinine levels in the previous 12 months predicted both rapid and severe eGFR decline at 12 months.
“[This] study demonstrates a role for [machine learning] models to predict rapid decline in eGFR,” the researchers wrote. “With the association of rapid eGFR decline with mortality in SCD, [machine learning] may play an important role in identifying patients at high risk for progressive kidney disease as early as 6 months in advance.”
“More studies are required to further evaluate [machine learning] models in SCD‐related kidney disease,” they wrote.