Grant Furthers Study of Machine Learning to Predict Kidney Decline
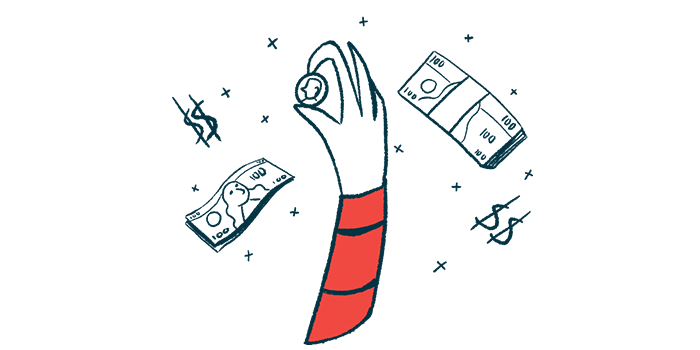
Scientists Kenneth Ataga, MD, and Santosh Saraf, MD, have received a $3.2 million grant to continue investigating the potential of machine learning as a method to help identify sickle cell disease (SCD) patients at high risk of developing progressive kidney disease.
The grant awarded by the National Heart, Lung and Blood Institute, part of the National Institutes of Health (NIH), will fund the research project “Predicting Progression of Chronic Kidney Disease in Sickle Cell Anemia Using Machine Learning Models (PREMIER)” for five years.
“We are grateful to the NIH for this award,” Ataga, director of the Center for Sickle Cell Disease at the University of Tennessee Health Science Center (UTHSC), said in a university press release.
Saraf, a co-principal investigator in the project, is an associate professor in the department of medicine at the University of Illinois in Chicago.
SCD patients have a higher risk of developing chronic kidney disease and experience fast kidney function decline. That’s why identifying patients at high-risk of kidney disease early on in the course of their illness is key to promptly begin interventions to slow kidney function decline.
Machine learning is a type of artificial intelligence that uses algorithms and statistical models to identify patterns, make predictions, and establish connections between multiple variable factors.
Ataga and his team have shown recently that machine learning tools can help identify SCD patients at high risk of progressive kidney disease as early as six months in advance.
Specifically, the machine learning analysis incorporated data from blood parameters, including the estimated glomerular filtration rate (eGFR), and immature red blood cell (reticulocyte) count, as well as blood pressure, stroke history, diabetes, body weight, age, sex, and medication use. Of note, eGFR is a kidney function measure based on the amount of creatinine — a waste product normally filtered by the kidneys and a standard marker of kidney function — in the blood
In the new project, Ataga and his team will conduct a prospective, multicenter study to continue assessing the potential of machine learning tools to accurately predict the risk of kidney disease progression in SCD patients.
“This award will allow us to use machine learning techniques to build a predictive model for progression of chronic kidney disease in adults with SCD. Early identification of these patients is necessary to modify known risk factors and initiate targeted therapies in hopes of increasing their life expectancy,” said Ataga.